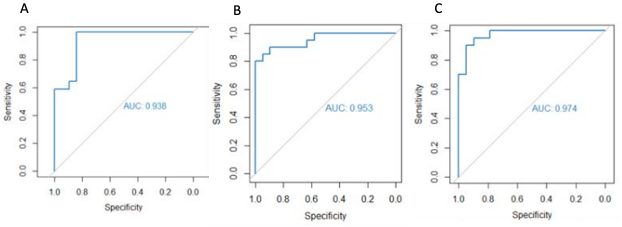
“Combination protein biomarkers predict multiple sclerosis diagnosis and outcomes.”
Eleftheria Kodosaki, et al. – UCL Institute of Neurology. Under review at Journal of Neuroinflammation.
Background: Serum biomarkers for MS still generally lag behind those in cerebrospinal fluid (CSF) for their diagnostic potential.
This Study: To determine the most effective testing strategy for detecting MS, Kodosaki and colleagues tested various combinations of serum and CSF biomarkers, seeking to distinguish 77 patients with MS from 80 controls who had CSF collected for reasons other than suspected demyelinating disease. Using serum markers alone, they found that an optimal model using osteopontin, the complement proteins iC3b and Factor B, and monocyte chemoattractant protein 1 (MCP-1), achieved an area under the receiving operator curve (AUC) of 0.94 on training data and 0.91 on testing data. These results were similar to those using the optimal model for CSF (5 markers; training, AUC = 0.96; testing, AUC = 0.94). For predicting time to next relapse, the optimal serum model (6 markers) showed the same level of concordance as the optimal CSF model (6 markers), and the optimal serum model for predicting time to EDSS 6 (4 markers) was slightly superior to the optimal CSF model (4 markers).
Bottom Line: Combining multiple serum biomarkers yields assays that are comparable in diagnostic performance to those using CSF, potentially enabling the use of blood tests for diagnosing MS. These findings need to be validated with comparisons using controls who are suspected of having demyelinating disease, but with an MS diagnosis subsequently ruled out, as this would represent the overall cohort being examined with these assays in the clinic.